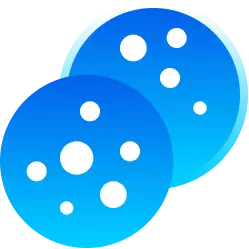
We Use Cookies!!!
We use cookies to ensure that we give you the best experience on our website. Read cookies policies.
In the age of big data, the ability to analyze and make sense of vast amounts of information is more important than ever before. While text and image data analysis have received a lot of attention, audio data analysis is becoming increasingly valuable.
As audio data is all around us, from speech recordings to music tracks, it has the potential to provide valuable insights about everything from consumer sentiment to market trends. However, in order to fully unlock the power of audio data, it is necessary to apply a process known as an audio annotation.
Furthermore, as smart speakers, voice assistants, and other voice-activated devices proliferate, the need for precise and trustworthy speech recognition will only grow. This makes audio annotation an essential tool for businesses and researchers looking to extract insights from audio data.
It is worth noting that according to a report by MarketsandMarkets, the global speech and voice recognition market is expected to grow from $9.4 billion in 2022 to $28.1 billion by 2027, representing a compound annual growth rate of 24.4%. The audio annotation will be the primary process here!
In this comprehensive guide to audio annotation, we will explore the various types and techniques of audio annotation, as well as its numerous use cases and benefits. From machine learning algorithms to automatic speech recognition tools, we will examine the latest trends and developments in audio annotation and provide practical advice for getting started with this powerful data annotation process.
Let’s start with understanding audio annotation first!
Audio annotation is the process of adding metadata or labels to an audio file to make it more easily searchable and analyzable. The audio annotation includes adding ground truths such as speaker identification labels, language, emotion, sentiment, background noise, music genre, and more for different use cases.
Audio annotation is a specific type of data annotation that is specialized for audio data. Through audio annotation, we are uncovering significant features stored in audio files, like what is being said, which speaker said what, how long each speaker speaks, emotions, intents, acoustic features, and whatnot!
With speech AI, our approach is to create human-like machines, and in order to train these machine learning models to understand real-world speech, we have to extensively train these speech models with unbiased and diverse speech datasets.
The goal of audio annotation is to create a structured and annotated speech dataset that can be used for various applications such as automatic speech recognition (ASR), natural language processing (NLP), emotion detection, and audio search engines.
These training datasets are collections of raw speech and ground truth information that the model can understand and use to find some patterns. So audio annotation is the process of adding specific ground truths in the form of labels and information to the raw audio data.
Unlike any other type of data annotation, audio annotation can be more complex and nuanced as it involves multiple layers and is affected by various parameters like accents, dialects, background noise, and so many other things.
Let’s understand why audio annotation is so important!
You'll have to admit that there are many different types of audio and speech data around us. The reason is that speech is the easiest and most convenient mode of communication compared to text or any other medium.
Speech and artificial intelligence advancements have resulted in a significant increase in the use of speech to access technology and services across domains.
The majority of us have smart speakers in our homes, and according to Google, 72% of people who own a voice-activated speaker use it as part of their daily routine, while 41% of voice assistant users say they feel like they are talking to a friend or another person. I guess it's the same for all of us.
Searching for anything on search engines has never been so easy. We all can now access the world of information with a single speech query while doing anything like driving or walking. Reports suggest that 50 percent of the United States population uses voice search features daily.
In order to make these machine learning models so robust that they can comprehend and revert back with the appropriate information, we need audio annotation as a fundamental process.
Another exciting field is speech data indexing! Every day, 271,330 hours of audio-video content are created on YouTube alone. The same is true for all audio and video platforms.
These audio and video files are referred to as "dark data" because we cannot search for specific words in them as we can do with text. In order to build AI models that can get the most out of this dark data, audio annotation is a must.
Even in the domain of customer service and call centres, AI enables us to analyze the conversations between service executives and customers. With the help of speech-enabled AI, we can analyze customer behaviour and sentiment and take the necessary actions. Audio annotation is crucial in this as well.
Audio is used as a primary mode of communication in many industries, including healthcare, customer service, education, and entertainment, and audio annotation can be a step toward revolutionizing how consumers interact with these businesses.
Now let’s explore different types of audio annotation techniques in detail!
Depending on the application and the specific information that needs to be extracted from the audio data, different types of audio annotation are used. Here are some of the most common types of audio annotation:
Transcription, also known as "speech-to-text," is a type of audio transcription in which all spoken words are converted into written text. Through transcription, we provide a detailed understanding of each word spoken in the audio file. This word-to-word transcription is referred to as “verbatim transcription” and is often used to train an automatic speech recognition (ASR) model.
This transcribed text and corresponding audio file can be used as a training dataset for automatic speech recognition models. A quality training dataset not only contains the word-to-word text of the audio, but along with that, it contains various other information like speakers, filler words, Personal Identifiable Information (PII), timestamps, and many other features that are present in the audio file. Depending on the application, these features may change.
There are many industries where transcription can be useful, especially those where speech and language are important. The following are some of the most typical uses for transcription:
Automatic Speech Recognition:
Transcribed audio can be used to train speech recognition software, which can improve the accuracy of automated transcription and voice-to-text technology.
Language Translation:
Transcription can be used to transcribe audio content in one language and then translate it into another language using AI-powered language translation tools. In the multilingual business world, where communication is crucial, this technology is especially helpful.
Content Indexing:
Transcription can be used to automatically index audio and video content, making it easier to search for and find specific information. This technology is useful for large enterprises with vast amounts of audio and video content.
The process of identifying specific speakers within an audio recording is known as speaker identification. Finding out who is speaking at any given time in the recording is the aim of speaker identification. This type of annotation is particularly useful in situations where multiple speakers are present in a recording, such as in conference calls, focus groups, and interviews.
Speaker identification and speech transcription are both similar types of audio annotation, but they serve different purposes.
Speaker identification is the process of identifying who is speaking at a given point in an audio recording. It is often used in applications such as forensic investigations, where identifying the speaker can be important for solving a crime, or in call centres, where it may be used to identify individual agents and track their performance.
Speech transcription, on the other hand, is the process of converting speech in an audio recording into text. It is often used in applications such as virtual assistants, where users can speak to their devices in natural language and receive responses in real time.
While both speaker identification and speech transcription involve processing audio data, the algorithms and techniques used for each task are quite different. Speaker Identification requires the ability to distinguish between different voices and identify them as belonging to specific individuals, while Transcription requires the ability to accurately transcribe speech into text while accounting for variations in accent, pronunciation, and other factors. In many cases, speaker identification is part of speech transcription.
Speaker Diarization:
Speaker identification can be helpful in training speaker diarization models that can segment a multi-speaker audio recording into individual segments, each corresponding to a particular speaker. Speaker diarization can be useful in applications such as call centre analytics, where it can help companies identify patterns in customer interactions and improve customer service.
Voice-enabled Personal Assistants:
Speaker identification is a technique used by many voice-enabled personal assistants, including Amazon Alexa and Google Assistant, to customize their responses based on the identity of the speaker. This can include personalized music playlists, reminders, and other features.
Emotion identification is a type of audio annotation that involves the identification and analysis of the emotional state of a person based on their speech or vocal cues.
Emotion identification annotation is a process of labelling audio data based on the emotions conveyed by the speaker. To perform this type of annotation, annotators listen to the audio data and mark the sections of the audio where the speaker's emotions change or exhibit a specific emotion, such as anger, happiness, sadness, or surprise.
There are various methods for carrying out emotion recognition annotation. One method is to categorically label each segment of the audio data using one of the predefined emotions that the annotator chooses from a list of options. An alternative method is the dimensional labeling system, in which the annotator uses a continuous scale to rate the intensity of various emotions.
Building models that have the potential to revolutionize how we interact with machines and enable more natural and intuitive communication can be aided by emotion identification annotation. Trained models can be used to classify the emotional state of new recordings in real-time, providing valuable insights into how people are feeling and enabling more personalized and responsive interactions with machines.
Personalized Care:
In healthcare, emotion recognition can be used to monitor patients’ emotional states and provide an early diagnosis for mental health disorders. It can also be used to analyse the emotional state of patients during therapy sessions. Emotion identification annotation can help in training such models.
Customer Service:
Emotion recognition in customer service can be used to examine customers' feelings as they communicate with voice bots, call centres, and other platforms for customer service. This can assist businesses in understanding the needs of their clients and offering specialized support.
Sentiment identification audio annotation is a type of audio annotation used to detect and analyze emotions, opinions, and attitudes expressed in spoken language. It entails annotating audio data with labels that express the speaker's attitude, such as positive, negative, or neutral. Several contexts, such as media monitoring, political speeches, and customer service interactions, can benefit from the use of sentiment identification audio annotation.
Machine learning models can be trained to automatically detect sentiment in audio recordings using the annotated speech data on sentiment identification.
One of the challenges in sentiment identification is dealing with the nuances of human language, such as sarcasm, irony, and cultural context. Additionally, the tone, pitch, and other acoustic characteristics of the speaker can affect the sentiment. In order to ensure the quality of the annotated data, it is crucial to have knowledgeable annotators who can accurately capture these subtleties.
Looking from the top, both of these audio annotations, sentiment identification and emotion identification look very similar in nature. But there is a subtle difference in the focus attribute.
In sentiment identification, we are interested in labelling the speaker's overall sentiment or attitude toward a specific topic. Whereas in emotion identification, we are more interested in annotating different emotions and the intensity of these emotions in an audio file's speaker.
Both of these audio annotation techniques are crucial for analyzing audio data, but they require different approaches and methods of annotation.
Customer service:
Sentiment identification can be used to analyze customer support calls and customers’ feedback and determine how customers feel about a business or product, which can help businesses develop their products and customer service.
Media Evaluation:
To better understand public sentiment toward current events and news topics, sentiment identification can be used to analyze news broadcasts, podcasts, and other media content.
Both the terms "acoustic event identification" and "sound event identification” audio annotation refers to the same thing in essence, and the terms can be used interchangeably. This audio annotation involves identifying and categorizing specific sounds or events within an audio recording.
The goal of this annotation is to detect and label the presence of specific sound events in an audio recording. This can include recognizing sounds like dog barks, car horns, sirens, and musical instruments, among others.
To perform sound event identification annotation, the annotator listens to the audio recording and labels the specific sound events present in the recording. Machine learning models are then trained to automatically recognize these sounds using the annotated data.
Smart Home Devices:
Sound event detection can be used in smart home devices to recognize different sounds, such as doorbells, smoke alarms, or baby cries, and trigger appropriate responses, such as sending a notification or turning on the lights.
Public Safety:
Sound event identification can be used to identify specific sounds, like gunshots or screams, and notify authorities in case of an emergency in public places like train stations or airports.
Healthcare:
Monitoring and analysis of sounds like breathing patterns, coughs, and snores can be done using sound event identification in the medical field to help diagnose and treat respiratory diseases and sleep apnea.
Automotive Industry:
In the auto industry, abnormal sounds that could be signs of problems or defects can be monitored, diagnosed, and detected using sound event detection.
The process of locating and labeling particular speech segments within an audio recording is known as "speech labeling audio annotation." In addition to identifying the spoken words or phrases, this also entails determining the speaker's gender, age, accent, tone, and emotional state.
Speech labeling can be thought of as a collection of different audio annotations, such as speaker identification, emotion identification, sentiment identification, or transcription. Applications like speaker identification, audio segmentation, and speech recognition can all benefit from this kind of annotation.
Transcription Tools:
Speech labeling can be used to build robust transcription tools that provide accurate and reliable transcription services for a variety of industries, such as legal, medical, and academic.
Voice Bots and Virtual Assistants:
Speech labeling can be used in the training process of voice bots and virtual assistants.
Audio classification is the process where human annotators listen to and categorize audio data into specific classes or categories based on their features or characteristics. It involves identifying different types of sound present in the audio and labeling them with descriptive tags or categories that represent their content.
Annotators might, for instance, listen to park recordings and label various audios with terms like "birdsong," "children playing," "dogs barking," or "footsteps on gravel." These annotations can then be used to train a machine learning model to automatically recognize different types of environmental sounds.
Another case may be where the annotator may listen to various audios of human intersecting with voice assistant and tag the user response to assistant to one of the predefined tag like, “Yes”, “No” or “Maybe.”
Voice Assistants
Audio classification can be very helpful in training voice assistants in identifying, differentiating, and understanding the human voice and other passing sounds.
Automatic Text-to-Speech model
Audio classification can be used in training an automatic speech transcription model to identify and label different sounds and transcribe the human voice accurately.
Speech act identification is a type of audio annotation that involves identifying the intention or purpose behind a spoken utterance. It is also referred to as “intent identification.” In this annotation, we are identifying the intent of the speaker and labeling the audio based on that.
For example, a speech act can be classified as a question, a statement, a command, or a request. If the speaker says, "I need help with my account," it can be labeled as a request. Similarly, if the speaker says, "Can you tell me more about your pricing plans?" it can be labeled as a question.
This annotated training dataset can be used to train speech act classification models that can automatically identify the intent of the user and serve them with appropriate answers.
Customer Service Voicebot
Speech-act identification can be helpful in training customer service voice bots that can identify the user's intent and provide accurate service, as discussed above.
Language identification audio annotation is the process of labelling audio data with the language that is being spoken in it. It can be used in order to create a training dataset for training spoken language identification models.
Language identification audio annotation can be challenging due to factors such as the presence of multiple languages in the audio data, the use of dialects and accents, and the presence of background noise.
However, accurate language identification is essential for many AI applications that require language-specific processing. It is an essential task for applications like speech recognition, machine translation, and multilingual content indexing.
Automatic Speech Recognition Model Selection:
Language identification can be useful in training models that can automatically identify audio language, and based on this output, appropriate speech recognition models can be adopted.
As now understood, audio annotation is essential for speech-based AI models. There is no way to train supervised speech models without a quality training dataset and to prepare a quality dataset, audio annotation is a must!
However, there are some difficulties that must be overcome when working on any audio annotation project, as listed below:
For audio annotation, we have to rely on manual work. There is no doubt that there are some AI models that can automatically transcribe or label audio data, but accuracy is not up to the mark yet. These AI models are not yet fully trained to tackle different nuances of speech data.
So even after using these AI tools, we still need humans to ensure quality. In our experience sometimes the sum of the time spent reviewing and editing AI-labeled data can be more time-consuming than doing it manually. Audio annotation can be a time-consuming and tedious task.
To annotate or label speech data, we need native language experts. As speech data can be complex in terms of voice, noise, language, and complexity, we need experienced people who can understand the nuance and label it accurately.
While we are building speech AI solutions for any specific domain, we have to train the model on specific domain speech data. To annotate such domain-specific speech data, we need subject matter expert annotators for it.
It is essential to have a tool that allows users to perform all of the necessary tasks on audio files, such as segmentation, transcription, speaker identification, filler words, speech labelling, intent annotation, and sentiment annotation. It is also very important that these tools provide JSON output in the required format in order to carry out an easy model training process.
It is difficult to find such a robust audio annotation tool that is also easy to use for annotators.
As the entire AI ecosystem is growing at a rapid pace, it is possible that we will need to work on different types of audio annotation projects in multiple languages simulteneously. In that case, we'll have to collaborate with hundreds, if not thousands, of people who speak different languages.
At this time, we require an expert crowd management team as well as crowd management software that can assign and unassign projects, track progress, and provide you with final output in the required format in the shortest amount of time.
To build robust AI, we have to feed a diverse speech dataset, and in doing so, we also need some data with edge use cases, in which there may be heavy background noise, bilingual speakers, or non-native speakers. Annotating such audio files can be complex.
One of the biggest challenges for audio annotation is the potential for biased annotators. When performing the annotation task, annotators may bring their own biases and viewpoints to the table, which may result in mistakes or inconsistencies. It can be challenging to ensure that these biases do not permeate the dataset.
Annotation guidelines are the crucial channel for conveying annotation requirements and processes across different annotator teams. Preparing accurate guidelines for annotation and training annotators on that can be very complex. Without this, we cannot ensure consistent annotation.
Untrained and immature annotators may make mistakes in annotation, such as incorrect transcription, inaccurate segmentation, mislabeled data, etc. Reviewing a large dataset entirely can be complex and time-consuming, so it can lead to a low-quality dataset.
You must be wondering how to cope with these challenges in a rapidly changing AI eco-system.
When it comes to working on an audio annotation project, there are mainly two approaches: in-house or partnering with third-party experts like FutureBeeAI.
The in-house approach involves building an annotation team within the organization. This team is in charge of choosing the tools for the annotation process, creating the annotation guidelines, and carrying them out.
On the other hand, partnering with an expert involves outsourcing the annotation process to a specialized service provider. In this case, the service provider will help you finalize the requirements, create guidelines, onboard annotators, train them, and carry out annotations.
Similar to any other data annotation process, audio annotation is also an expertise-driven and complex process. Building your own in-house team can be a good decision in case of
But when this is not the case. When you want to focus on building an AI solution and want to scale it quickly it can not be the best decision for you as
Our experience working with some of the leading AI organizations gives us the understanding that each one of these challenges can have extreme implications on quality, timeliness, and budget. That eventually hurt the purpose of building robust AI solutions.
We understand that quality in terms of data and scale in terms of the process is an ideal match for any AI organization working on annotation projects. The approach to mitigate these challenges can be found in the PPT formula; people, process, and tools.
People: Leverage Expertise
In order to create high-quality training datasets to train our speech model, human intervention is required from data collection to audio annotation. There are two layers of human-in-the-loop for any data annotation project, and nothing is different for audio annotation as well.
Annotators:
As discussed earlier, we need native-language expert annotators who can understand the nuances and features of the audio data and easily annotate it with quality. With the FutureBeeAI crowd community, you can leverage the 10000+ people from all across the globe in almost all languages and work on your AI objective. Their experience working with us on various audio annotation projects qualifies them to handle your needs accurately.
Project and Community Management Team:
Training, handling, and transferring your vision to annotators can be a challenging task, but our expert in-house project management team can efficiently help you with end-to-end solutions.
To efficiently deliver the expected result, we need standard operating procedures (SOPs) for each step of the annotation process. With our experience of serving leading clients in the ecosystem, we have developed SOPs that work almost all the time.
Before beginning any project, each stage, from understanding the use case and requirements to creating guidelines, finding and onboarding a crowd, project management, quality evaluation, and delivery, requires a detailed plan. Each of these major stages contains many important sub-stages and can cause continuous back and forth with the client, which can increase the overall timeline and budget. With our time-proven, experience-driven process, this can be easier than ever before.
Although there are already plenty of tools available in the market, some of them are paid and some are open source, but a comprehensive tool that is easy to use for annotators is lacking.
That’s why we are coming up with a state-of-the-art audio annotation tool that can handle all your audio annotation-related requests. As mentioned earlier, reviewing and identifying small annotation mistakes can be complex, so with our auto-validation feature, we can ensure annotation consistency and reduce errors easily.
So, I think that after such an extensive discussion, you must agree that delegating your audio annotation task can be a decision that can define the success of your speech AI innovation. What is more crucial is to choose an appropriate partner for this. Feel free to contact us and let us prove that we are the one. We would love to discuss how we can bring quality work to the table for you!