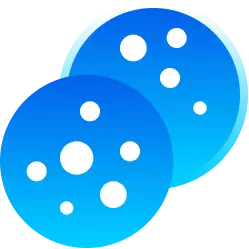
We Use Cookies!!!
We use cookies to ensure that we give you the best experience on our website. Read cookies policies.
As the use of artificial intelligence and machine learning continues to grow, companies are increasingly turning to speech and image recognition AI/ML models to improve their products and services. However, creating these models can be a complex and time-consuming process, requiring the selection of appropriate training data and a reliable data annotation company.
Outsourcing training data collection and data annotation for AI/ML models can be a valuable strategy for businesses looking to create high-quality speech and image recognition systems. By partnering with a specialized company, businesses can access a team of experienced annotators and a diverse pool of data, ensuring that their AI/ML models are trained on a broad and representative set of examples.
But with so many companies offering data annotation services, how do you choose the right one for your business?
In this blog, we will explain the key factors to consider when selecting a training data provider and a data annotation company to ensure the success of your AI/ML model.
First, let's define what training data and data annotation are.
Training data is the input data that is used to train an AI/ML model. This data is labeled and categorized, which helps the model learn to make predictions and decisions based on the data it receives. Data annotation, on the other hand, is the process of labeling and categorizing the training data. This is typically done by human annotators who have expertise in the relevant field. Sometimes it can also be done by algorithmic annotation tools which are then followed by manual annotation according to needed accuracy.
The first step in selecting training data is to determine the specific purpose and goals of the AI/ML model. This will help identify the type of data that will be most relevant and effective for training the model. For example, if the goal is to create a speech recognition model for a specific language, the training data should include a diverse range of audio recordings in that language.
Once the purpose and goals of the model have been established, the next step is to evaluate the quality and quantity of the training data. High-quality data is essential for creating accurate and effective AI/ML models, so it is important to ensure that the data is relevant, diverse, and free of errors or inconsistencies. It is also important to consider the quantity of data, as larger datasets can help improve the performance and accuracy of the model.
After selecting the appropriate training data, the next step is to choose a reliable data annotation company. Data annotation involves labeling and categorizing the training data, which is a critical step in the AI/ML model creation process. The quality of the data annotation can significantly impact the performance and accuracy of the model, so it is important to choose a company with a proven track record of providing high-quality data annotation services.
When choosing a data annotation company, it is important to consider several key factors, including their experience and expertise in the specific field, the quality of their work, and the accuracy and consistency of their annotations. It is also important to consider their turnaround time and the cost of their services, as well as their ability to provide customized solutions to meet the specific needs and goals of the project.
Before getting into factors, let’s understand why one should consider outsourcing annotations over in-house.
There are several key differences between outsourcing data annotation and handling it in-house. These differences can impact the cost, efficiency, and quality of the data annotation process.
Outsourcing data annotation can often be more cost-effective than handling it in-house, particularly if the volume of data to be annotated is large. This is because outsourcing allows you to take advantage of economies of scale and tap into a pool of skilled annotators who are already trained and ready to work. In contrast, handling data annotation in-house requires you to invest in training annotators, which can be time-consuming and costly. Additionally, you may need to pay for additional resources such as software and hardware, which can further drive up costs.
At an efficiency level, outsourcing can be more efficient because you are able to tap into a pool of skilled annotators who are able to work on the task quickly and accurately. This can help you complete the data annotation process more quickly, allowing you to move on to other tasks sooner. In contrast, handling data annotation in-house may be less efficient if you do not have a dedicated team of annotators or if your annotators are not as experienced or efficient at the task.
Accessibility for a large, skilled workforce can result in higher-quality annotations. This is because experienced annotators are able to produce more accurate and consistent annotations, which can be critical for ensuring the quality of the data. In contrast, handling data annotation in-house may result in lower-quality annotations if you do not have access to a team of skilled annotators or if your annotators are not as experienced.
Now, let's discuss these key factors to consider when selecting data annotation services.
The quality of data annotations is critical to the success of any machine learning project. Poorly annotated data can lead to poor model performance and incorrect results. Therefore, it is important to choose a data annotation service provider that has a proven track record of producing high-quality annotations. This can be assessed through reviews, case studies, and sample work.
There are several metrics that can be used to measure the quality of data annotations. Some of the most common ones include Inter-annotator agreement, annotation accuracy, annotation speed and annotation completeness.
As mentioned earlier, data annotation is the process of labeling and categorizing the training data. It is crucial to select a data annotation company with expertise in the relevant field and can provide accurate annotations. For example, if you are building a medical image recognition model, it is important to select a data annotation company that has experience annotating medical images and can accurately identify and label various medical conditions and abnormalities.
Annotation expertise and accuracy metrics include: Training, experience, and attention to detail.
Training data often contain sensitive information, such as personal information or confidential business data. It is crucial to select a data annotation company that has robust data privacy and security measures in place to protect your training data. This includes measures such as encrypted data storage, secure data transfer protocols, and strict access controls.
As your AI/ML model grows and evolves, you may need to add more training data or make changes to the existing data. It is important to select a data annotation company that can scale and adapt to your changing needs. This includes quickly adding more annotators, supporting different annotation formats, and handling large volumes of data.
Your chosen strategic annotation partner is the best option to consider if they also have their own annotation tools for the necessary format. Because it adds an advantage of trust factor over other outsourcing partners into your consideration. But be sure to check tool specifications and features before signing up, as it might affect other factors based on the tool operating type(Manual, semi-automatic and automatic).
Training data and data annotation can be a significant investment, so it is important to consider the cost and turnaround time when selecting a data annotation company. Be sure to compare prices and turnaround times across different companies to find the best fit for your budget and timeline.
The larger the volume of data that needs to be annotated, the higher the cost will be. This is because more time and resources will be required to complete the task.
Data that is more complex or requires specialized expertise will typically cost more to annotate. For example, medical images or natural language processing data may require more specialized annotators, which can increase the cost.
Different types of annotation can require different levels of expertise and take different amounts of time. For example, image classification may be less complex and take less time than object detection, which requires more detailed annotations.
In addition to these factors, it is also important to consider the type of data annotation services offered by the company. Some agencies may offer manual data annotation, which involves trained experts manually labeling and categorizing the data, while others may offer automated data annotation, which uses algorithms and machine learning techniques to annotate the data automatically. Each approach has its own strengths and limitations, so it is important to choose a company that offers the type of data annotation services that are most appropriate for the specific project.
We at FutureBeeAI have extensive experience and expertise in structuring accurate data annotation and labeling services for speech and image annotations and other data formats for AI/ML models. Our team of trained experts uses a combination of manual and automated data annotation techniques to ensure the accuracy and consistency of our annotations.
FutureBeeAI offers a range of data annotation services, including text and image annotation, audio and video annotation, and NLP and NLU annotation, to help our clients create accurate and effective AI/ML models. Our services are customized to meet the specific needs and goals of each project, and we offer competitive pricing and fast turnaround times to help our clients achieve their goals.
If you are looking👀 to create a speech or image recognition AI/ML model, contact us today.
🔗 Helpful Resources
https://www.futurebeeai.com/image-annotation
https://www.futurebeeai.com/audio-transcription
https://www.futurebeeai.com/text-annotation