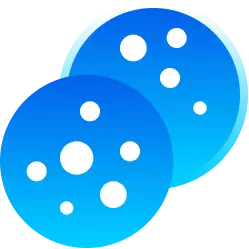
We Use Cookies!!!
We use cookies to ensure that we give you the best experience on our website. Read cookies policies.
AI is revolutionizing industries across American and European continents, rapidly integrating into every conceivable use case. But in a diverse country like India, with over 140 crore people and 22 official languages along with countless regional dialects the need for AI solutions tailored to our unique demographic is more critical than ever. India stands on the brink of an AI-driven transformation that can significantly improve the lives of millions. To build AI models capable of understanding and serving such a vast and linguistically diverse population, we must prioritize the creation of robust and high-quality datasets. The effectiveness and reliability of AI hinge on the data it's trained on. In a country as diverse as India, compromising on data quality is not an option. As AI experts rightly say, "Data is the only moat," and in the context of Indian languages, this moat must be deep and wide to truly make an impact.
So, if you are building an India specific AI solution then you are on the right track to find out high quality speech datasets for Indian languages. In this blog we will explain different types of speech datasets for Indian languages. Let’s discuss them one by one.
In the diverse landscape of India, where numerous languages and dialects are spoken, call centers play a pivotal role in customer service across various sectors, from banking and telecommunications to healthcare and e-commerce. To develop AI-driven solutions that can understand and process conversations in Indian languages, high-quality call center speech data is essential. This data, consisting of recorded interactions between agents and customers, is the cornerstone for building robust AI models that can handle the linguistic diversity and complexity of the Indian market.
We FutureBeeAI provide this type of data in most Indian languages and cover most of the industries. With our diverse team of linguists across India we are able to record and collect such data at scale.
Our contact center speech data have content specific to use cases. In these datasets we have standard and local both terminologies used in the calls.
●Multiple Languages: The dataset should encompass a wide range of Indian languages, such as Hindi, Tamil, Bengali, Telugu, Marathi, Kannada, Maithili, Bodo and others.
●Regional Dialects Within these languages, it’s crucial to include variations in dialects to capture the true linguistic diversity of the country.
●Code-Switching: In many conversations, speakers switch between languages, especially between English and a regional language. The dataset should reflect this common practice.
●Customer Inquiries: Data from calls where customers ask about product details, services, and account information.
●Complaint Handling: Conversations involving customer complaints and their resolutions.
●Sales and Promotions: Interactions where agents are explaining offers, promotions, or upselling products.
●Support Calls: Technical support or troubleshooting conversations that may involve more detailed and complex language.
●Feedback and suggestion: Conversations where customer sharing suggestions and feedback to improve solutions.
●Speaker Labels: Each part of the conversation is labeled with speaker tags, such as "Agent" and "Customer," to identify the source of the speech.
●Transcriptions: Accurate manual transcriptions of the spoken language, capturing not just the words but also the nuances like pauses, and filler sounds.
●Sentiment Annotation: Labeling the emotional tone of the conversation (e.g., positive, negative, neutral) to help AI models detect customer satisfaction or frustration.
●Entity Recognition:: Identifying and labeling key entities such as product names, service terms, dates, and numbers mentioned during the conversation.
●Intent Classification: Annotating the purpose or intent behind each customer query, such as inquiry, complaint, feedback, or request for service.
●Silence and Overlaps: Marking periods of silence, overlaps in conversation, or background noise that could affect the clarity of speech recognition.
●Call Duration: Information about the length of the call, which can be used to understand conversation complexity.
●Geographical Data: Location-based tagging to identify regional language variations and accents.
●Call Type: Categorization of calls by their nature, such as inbound or outbound, customer service, or sales.
●We record these datasets in loss-less format, “wav”.
●These datasets are recorded at different sample rates.
●These datasets are available in mono and stereo channels.
The use of annotated call center speech data in Indian languages is crucial for developing AI models that can perform tasks like automated transcription, sentiment analysis, and intent recognition with high accuracy. Such models can then be used to enhance the efficiency of call centers, automate routine queries, provide agents with real-time assistance, and even predict customer and agent behavior based on historical interactions.
With accurate speech data, AI systems can be trained to understand the subtleties of Indian languages, handle code-switching seamlessly, and respond to customers in a more natural and human-like manner. This leads to better customer experiences, reduced operational costs, and a higher overall satisfaction rate.
As voice assistants become increasingly integral to daily life, the importance of high-quality speech datasets in Indian languages cannot be overstated. These datasets are crucial for training voice assistants to understand and respond accurately to commands in a diverse linguistic landscape. Here’s an overview of the essential components and use cases for Indian languages speech datasets tailored for voice assistants.
In the automotive sector, voice assistants enhance driving safety and convenience by allowing drivers to control various vehicle functions through voice commands.
●Wake Words: Speech samples for activation phrases like "Hey Hyundai" or "Hello Ola" in multiple Indian languages.
●Navigation Commands: Data for commands such as "Navigate to the nearest petrol pump" or "What's the fastest route to Mumbai?" in languages like Hindi, Tamil, Telugu, and others.
●Entertainment Controls: Commands for media management, such as "Play Allu Arjun latest’ movie song" or "Change to FM 94.1."
●Vehicle Functions: Data for controlling settings like "Turn on the air conditioning" or "Open the sunroof."
●Emergency Assistance: Commands for urgent actions, such as "Call emergency services" or "Report an accident."
●Command Intent: Categorizing commands by their function, like navigation, media control, or vehicle settings.
●Contextual Data: Including environmental noise, such as engine sounds and traffic, to ensure the assistant can operate effectively in a moving vehicle.
Voice assistants in smart home devices enable users to manage their home environment through voice commands.
● Wake Words: Examples like "Hey Home" or "Hello Assistant" in various Indian languages.
●Home Automation Commands: Data for controlling devices such as "Turn on the living room lights" or "Set the thermostat to 22 degrees Celsius."
● Appliance Management: Commands for appliances like "Start the washing machine" or "Preheat the oven."
●Security Systems: Commands for managing security, such as "Arm the security system" or "Show me the front door camera."
Voice assistants in mobile devices, smart speakers, and other general-use products rely on a wide range of voice commands.
● Wake Words: Common phrases like "Hey Google" or "Hello Bixby" in multiple Indian languages.
●Command Data: Includes everyday interactions such as "Send a message to Priya" or "Play my favorite playlist."
●Information Queries: Commands like "What's the weather today?" or "Tell me the latest news."
● Task Management: Commands for setting reminders, managing calendars, and other personal tasks.
● Intent Detection: Categorizing commands into functions like messaging, media playback, or information retrieval.
● Contextual Variations: Including diverse linguistic styles and speech patterns across different Indian languages.
● Multi-Speaker Environments: Annotating interactions involving multiple users or background noise.
For voice assistants to be effective across India’s diverse linguistic landscape, the speech datasets must reflect the country's wide range of languages and dialects. Key aspects include:
●Language Coverage: Incorporating a broad spectrum of Indian languages and dialects, including regional variations and code-switching practices.
● Accent and Pronunciation: Training models to recognize and accurately process different regional accents and pronunciations.
●Contextual Relevance: Ensuring datasets capture real-world scenarios, including background noise and varying acoustic environments.
If you are building a voice assistant that can understand Indian languages then we can partner with you to provide all the required data. Apart from the above mentioned use case we can also record the data for custom use cases. Our datasets can be your moat to serve and scale with AI.
Scripted monologues in Indian languages are a crucial dataset component for a wide array of applications, particularly in AI and language technology. These datasets consist of pre-written speeches delivered in a structured and consistent manner across various Indian languages, including Hindi, Tamil, Bengali, Telugu, and many others. By using scripted content, developers can capture diverse linguistic patterns, regional accents, and pronunciation nuances, which are essential for training and fine-tuning speech recognition models and other AI systems.
●Linguistic Diversity: Incorporates a wide range of Indian languages and dialects, ensuring broad applicability across different regions of India.
●Controlled Speech Patterns: Provides structured and predictable language data, which is ideal for training AI models that require consistency in language input.
●Accent and Pronunciation Variations: Includes speakers from various regions, capturing the rich diversity of accents and pronunciations found across India.
General conversation speech data is an essential component for creating natural and effective AI models. This dataset comprises recordings of spontaneous, unscripted dialogues between speakers, capturing the natural flow of conversation in various Indian languages such as Hindi, Tamil, Bengali, Telugu, Marathi, and many others.
●Natural Language Usage: Captures the way people naturally speak, including the use of colloquial expressions, pauses, interruptions, and conversational fillers like "umm" or "ah."
●Diverse Interactions: Encompasses a wide range of conversational scenarios, from casual chats to more formal discussions, reflecting the versatility of everyday speech.
●Multilingual and Code-Switching: Often includes code-switching, where speakers switch between languages mid-conversation, a common practice in multilingual regions like India.
Multimodal data, which integrates both speech and image components, is a powerful resource for advancing AI and machine learning models. In the context of Indian languages, such datasets are particularly valuable for developing applications that require understanding and processing information from multiple sources.
●Speech and Image Pairings: Combines spoken language data with corresponding visual content, such as images of objects, scenes, or text. This pairing is especially important in creating rich, context-aware models.
●Linguistic Diversity: Includes speech data in various Indian languages, such as Hindi, Tamil, Bengali, Kannada, and others, paired with images that are culturally and contextually relevant.
●Cultural and Regional Contexts: Captures the unique cultural references, objects, and scenarios that are specific to different regions of India, ensuring that the data is representative of the population.
Developing models that can generate accurate descriptions of images based on speech input in Indian languages, aiding visually impaired users by providing verbal descriptions of visual content.
Creating systems that can answer questions about an image using speech input in a particular Indian language, enhancing educational tools and interactive learning experiences.
Enabling search engines or virtual assistants to retrieve images based on spoken queries, improving accessibility for users who prefer voice input over text.
Developing AR/VR applications that respond to spoken commands in Indian languages by displaying relevant images or scenarios, enriching user experiences in gaming, education, and virtual tours.
Supporting language learning apps that pair spoken words or phrases in Indian languages with corresponding images, helping learners associate words with visual representations and improve their vocabulary.
Documenting and preserving the rich cultural heritage of India by creating datasets that capture traditional objects, rituals, and practices through a combination of speech and images in regional languages.
All the above mentioned datasets are available on our datastore. FutureBeeAI has many years of experience to create such datasets and fulfill the data needs for India. You can visit our datastore for more details on each dataset.
Apart from our datastore for Indian languages, you can also rely on open source datasets made by the Indian government. The datasets made by the Indian government are good to start your AI initiative.
As all the governments are focussing on developing native AI models, Indian government have also taken many initiatives to build quality datasets for Indian languages and made then available to everyone.
AI4BHARAT and BHASHINI are two main organizations working on this initiative and collecting data from all regions of India. You can visit their sites for data and open source models.
We offer custom and industry specific speech, text, video and image training data collection services, as well as ready to use 200+ datasets for different industries. Our datasets include facial training data, driver activity video data, multilingual speech data, visual speech data, human historical images, handwritten and printed text images, and many others. We can also help you label, annotate and transcribe your data.
We have built some SOTA platforms that can be used to prepare the data with the help of our crowd community from different domains.
You can contact us for samples and platform reviews.