How are datasets curated for LLM training?
Data Collection
Data Annotation
Quality
Datasets for Large Language Model (LLM) training are curated through a process that involves:
- Data collection: Gathering text data from various sources, such as books, articles, websites, social media platforms and with the help of training data service providers.
- Data cleaning: Removing unnecessary characters, punctuation, and formatting.
- Tokenization: Breaking down text into individual tokens, such as words or subwords.
- Filtering: Removing duplicates, special characters, and irrelevant text.
- Preprocessing: Normalizing text, converting to lowercase, and removing stop words.
- Balancing: Ensuring the dataset is balanced in terms of topic, style, and genre.
- Anonymization: Removing personal information and sensitive data.
- Quality control: Human evaluation to ensure the dataset is accurate and relevant.
- Splitting: Dividing the dataset into training, validation, and test sets.
- Versioning: Keeping track of dataset versions and updates.
The goal is to create a diverse, representative, and high-quality dataset that enables LLMs to learn effective language understanding and generation capabilities.
What Else Do People Ask?
Related AI Articles
Browse Matching Datasets
Acquiring high-quality AI datasets has never been easier!!!
Get in touch with our AI data expert now!
Contact Us
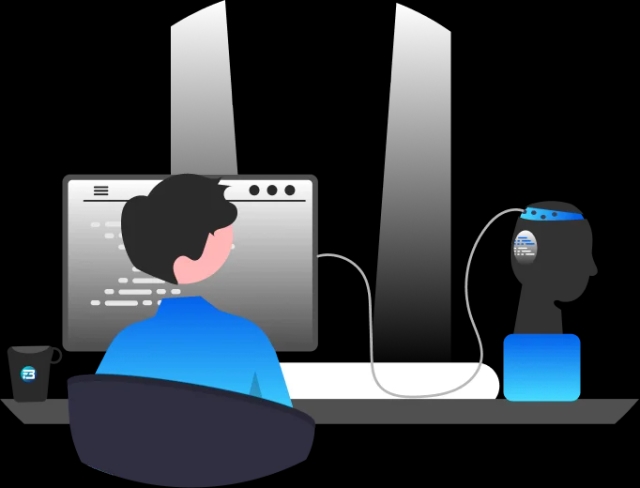