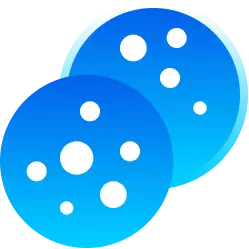
We Use Cookies!!!
We use cookies to ensure that we give you the best experience on our website. Read cookies policies.
Accents and dialects impact wake word recognition by introducing variations in pronunciation, speech speed, and intonation. A wake word, like "Alexa" or "Hey Siri," can sound different when spoken by individuals with various accents, such as American, British, or Indian English. These phonetic differences, such as vowel shifts or consonant substitutions, can confuse wake word recognition systems if they aren't trained on diverse speech patterns.
For example, in non-rhotic accents (like some British dialects), the "r" sound in words like "Siri" might be dropped, making the wake word harder for the system to detect. Similarly, speakers with faster speech rates or unique intonation patterns may blur the wake word, causing the system to miss the activation cue.
A significant factor is the training data used for these systems. Many wake word recognition models are trained on limited, standardized datasets, which may not adequately cover the variety of global accents and dialects. If a system hasn’t been exposed to diverse speech patterns during training, it may struggle to recognize wake words from users with unfamiliar accents.
To tackle these issues, we need to:
- Use diverse training data that includes multiple accents and dialects.
- Implement accent-specific models that cater to regional pronunciation.
- Adapt to users’ speech patterns over time, allowing the system to become more personalized.
Incorporating these strategies enhances wake word recognition, ensuring that voice assistants can respond accurately across a broader range of speech variations.
Get in touch with our AI data expert now!