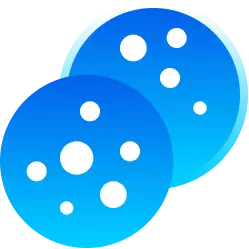
We Use Cookies!!!
We use cookies to ensure that we give you the best experience on our website. Read cookies policies.
Have you ever wondered how you get the results for product searches on an ecommerce or retail site or search engine? Sometimes, you might find yourself sifting through pages, trying different keywords, or refining your search criteria just to find what you're looking for. This journey reflects the intricate dance between you, the customer, and the concept of search relevance.
Search relevance is the backbone of our digital experiences, shaping how we discover information, products, and services online. It's not just about typing in a query; it's about finding precisely what we need quickly and effortlessly. But what exactly is search relevance, and why does it matter so much?
In essence, search relevance refers to the accuracy and appropriateness of search results in response to a user's query. Whether you're searching for a specific product, a piece of information, or even a local service provider, the relevance of the results determines how satisfied you'll be with your search experience. Let's delve deeper into the impact of search relevance on you, the customer and then we will discuss how you can become a data labeling freelancer for improving search relevance for ecommerce or retail.
Before we discuss what search relevance is, let’s understand how people actually search on the web to discover required results. People search on e-commerce websites in various ways, depending on their preferences, needs, and the design of the platform. Here are some common methods:
Users typically enter keywords or phrases related to the products they're looking for into the search bar. For example, if someone is searching for "Arabic Speech Data" they would type those words into the search field to find relevant products.
E-commerce websites often categorize products into different sections or categories, such as clothing, electronics, or home goods. Users can navigate these categories and subcategories to browse products in a more structured manner. Additionally, filters such as price range, brand, size, color, and ratings allow users to refine their search results based on specific criteria.
Some retail or e-commerce platforms incorporate recommendation algorithms that suggest products based on the user's browsing history, purchase history, or items they've added to their cart. These personalized recommendations can help users discover relevant products they may be interested in. Let’s say you are buying an iPhone from a website that can recommend relevant products that are useful for an iPhone user, for example iPhone cover, earphones, etc.
Some e-commerce websites offer visual search capabilities, allowing users to upload images or take photos of products they're interested in. The platform then uses image recognition technology to find visually similar products in its catalog.
With the increasing popularity of voice-activated virtual assistants like Amazon Alexa and Google Assistant, some e-commerce platforms support voice search functionality. Users can verbally request products or ask questions, and the platform will respond with relevant search results or information.
Overall, people can use any of these methods to search on the web to get desired results. So, as a customer you have multiple ways to get your desired results but also it makes it complicated for an e-commerce company to provide exact results as per your requirement. Let’s discuss the challenges ecommerce website faces.
All customers are not the same, right. Like the way I search is totally different and the way you search is totally different. That’s a big challenge for any online selling company to understand different queries for the same products. If these challenges are not overcome then companies can lose millions of dollars. Let’s see some of the challenges.
Users typically enter keywords or phrases related to the products they're looking for into the search bar. For example, if someone is searching for "Arabic Speech Data" they would type those words into the search field to find relevant products.
User queries may contain ambiguous terms or lack context, making it challenging to determine the user's intent accurately. For instance, a search for "apple" could refer to the fruit, the technology company, or even a brand of headphones. Understanding the context of the search and disambiguating ambiguous terms is crucial for delivering relevant results.
E-commerce platforms often have vast and diverse product catalogs, spanning numerous categories, brands, sizes, and specifications. Managing this complexity and ensuring that search results cover the entire catalog while remaining relevant to the user's query is a significant challenge. Additionally, new products are constantly being added, further increasing the complexity.
The quality and consistency of product data play a crucial role in search relevance. Inaccurate or incomplete product information, inconsistent categorization, or missing attributes can lead to irrelevant search results or difficulty in filtering and sorting products effectively.
So, these are some of the challenges ecommerce faces. Apart from users' queries, search relevance becomes more complicated because of having multiple products and all challenges can be solved with the help of proper machine learning algorithms and quality training data. To build a robust machine learning algorithm, we need quality training data and to build quality training data we need high quality data labeling.
Now we understand how people search and how its impact on the search relevance. Let’s discuss what search relevance is and how you can become a data annotator for improving search relevance.
Search relevance refers to the accuracy and appropriateness of search results in response to a user's query. In simpler terms, it's about how well the results match what the user is looking for.
Search relevance is crucial because it directly influences the user experience, efficiency of information retrieval, and overall success of online platforms. By ensuring that search results closely match user queries and intent, search relevance enhances user satisfaction, enabling individuals to find what they're looking for quickly and easily. This not only saves users time and effort but also increases the likelihood of achieving their goals, whether it's finding information, making purchasing decisions, or seeking recommendations.
Additionally, high search relevance drives higher conversion rates in e-commerce contexts, fosters customer loyalty and retention, and provides valuable insights into user behavior and preferences.
Poor search relevance leads to loss of business, as a customer when we get poor results we immediately move to other options. But the good thing is search relevance can be improved and as a freelancer you can save millions for business and improve customer experiences for many by becoming a data labeller.
To become a data annotator for improving search relevance you need to learn a few types of labeling.
Named Entity Recognition (NER) is a natural language processing task that involves identifying and categorizing specific entities mentioned within text data, such as names of people, products, organizations, locations, dates, and numerical expressions.
As a Data Labeler, your role is to identify and tag specific entities within product titles, descriptions, and customer reviews. These entities encompass brand names, product models, and pivotal features that enrich the tapestry of search engine understanding. By meticulously annotating these nuances, we empower search engines to sculpt more precise and tailored search results, catering to the discerning needs of users.
Sentiment Analysis is like having a mood detector for text. It's a technique where we figure out if a piece of writing is expressing positive, negative, or neutral feelings. By understanding the sentiment behind words, we can better understand people's opinions and reactions.
As a Data Labeler, your task involves categorizing customer reviews and feedback based on the sentiment they convey, whether it's positive, negative, or neutral. This process of sentiment annotation aids search engines in comprehending the opinions surrounding products, enabling them to prioritize search results in accordance with user sentiment.
By annotating each review, we can contribute to enhancing the search engine's ability to understand and respond to user feedback effectively.
Intent Classification is like deciphering the purpose behind someone's question. It's a technique used to understand what someone wants when they type or say something. By figuring out the intent behind a query, we can better help people find what they're looking for online.
As a Data Labeler, your duty is to categorize search queries into distinct intent categories, such as product research, price comparison, or purchase-ready. By annotating these queries, we can provide invaluable insight to search engines, allowing them to decipher the user's underlying purpose. Through this process, we can facilitate the delivery of highly relevant search results to meet needs and intentions of the user.
Image tagging is like giving labels or tags to pictures to describe what's in them. It's a way to help computers understand what's in an image so they can organize and retrieve them more easily. By adding descriptive tags, we make it simpler for search engines or other systems to find the right images when they're needed.
So, in image tagging your role is to give label products with their attributes, keywords and categories. This helps search engines to improve visual search capabilities.
Nowadays many retailers allow users to buy products with the help of voice based search and if you have tried it then you may have poor or irrelevant results. So, for improving voice based search relevance, search engines need to understand different pronunciation and nuances of different speech.
As a Data Annotator, here you can transcribe and translate users queries. This will help search engines to understand different pronunciation of the same words, different style of speech, different speed of speech and ultimately help improve voice based search results.
These are some of the types of annotation you should learn to become an annotator for improving search relevance. And these annotation techniques can be used for many other use cases.
We will continue this blog series to add more valuable insights and dig deep into the different annotation techniques.
FutureBeeAI is a leading organization providing data annotation and labeling services to improve data quality. You can join us in this journey and help build better AI for all. Join our community and learn about different data annotation techniques, their use cases and earn a fair amount of money.