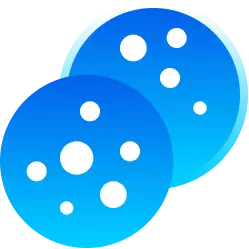
We Use Cookies!!!
We use cookies to ensure that we give you the best experience on our website. Read cookies policies.
Welcome to the era where your car isn't just a mode of transport; it's your conversational companion on the road. The automotive industry has ushered in a new wave of innovation, putting the power of voice assistants right at your fingertips, or rather, vocal cords.
Between September 2018 and January 2020, the use of voice assistants in cars among U.S. adults surged by 13.7%, reaching an impressive 129.7 million. Additionally, monthly active users witnessed a corresponding increase, reaching a notable 83.8 million during the same timeframe, as per research conducted by VoiceBot.ai.
However, perfecting this harmony between technology and the dynamic in-car environment comes with its set of challenges. In today’s blog, we are going to explore in-car ASR dynamics in detail.
In-car voice assistants are not only limited to listening to music or getting directions. It has made the life of driver and co-passengers life more comfortable, entertaining, and safe.
Music Selection and Control:
Drivers can effortlessly choose and control their music preferences with simple voice commands like “play the English rap songs”, which enhances the overall driving experience without taking their eyes off the road.
Capgemini predicts that 85% of consumers will be using a voice user interface for music and navigation by 2022.
Navigation and Directions:
In-car voice assistants provide seamless navigation, offering real-time directions and updates, ensuring a safer journey as drivers receive guidance without distraction.
Hands-Free Communication:
Voice assistants enable drivers to make calls, send texts, and manage communication without compromising safety.
Smart Home Integration:
Extend control beyond the car by integrating with smart home devices. Users can command their home systems, adjust thermostats, turn on lights, or even lock doors, all while driving.
Appointment Booking:
Voice assistants facilitate hands-free scheduling by allowing users to book appointments, set reminders, and manage their calendars, streamlining tasks while on the move.
According to Capgemini, by 2022 74% of consumers will be extremely comfortable using voice to book appointments and will prefer to use the in-car voice assistant to help.
Voice Search and Ordering:
Conducting searches for nearby amenities, restaurants, or services becomes effortless with voice-activated searches. Some systems even enable voice-driven orders and reservations.
Feedback and Reviews:
Users can provide feedback on various aspects of their driving experience through voice commands, contributing to the improvement of in-car systems and services.
Climate and Comfort Control:
Adjusting climate settings and ensuring a comfortable driving environment is made easier through voice commands, allowing drivers to focus on the road while making necessary adjustments.
Entertainment Management:
In-car voice assistants offer control over various entertainment features, such as adjusting volume, selecting radio stations, or even recommending podcasts and audiobooks.
Safety Alerts and Assistance:
Voice assistants can enhance safety by providing real-time alerts, weather updates, and emergency assistance, contributing to an overall safer driving experience.
In-car voice assistants are built on Automatic Speech Recognition (ASR) systems. ASR is a crucial component of voice recognition technology that converts spoken language into written text.
When a user speaks a command or asks a question, the in-car ASR system processes the spoken words, identifies the individual words or phrases, and converts them into text. This text is then used by the voice assistant to comprehend the user's intent and provide the appropriate response or action.
As we know the accuracy of the ASR model depends upon various factors including but not limited to acoustic environment, background noise, and lots of other features. So any regular ASR model trained with general speech data will not perform efficiently in an in-car environment.
To ensure accuracy and seamless use of in-car voice assistants for all the use cases discussed above we need to matchlessly train the speech recognition model on the custom speech dataset.
So in-car speech recognition models are different in terms of the speech dataset on which they are trained. Now let’s discuss some of the challenges that are there while training an in-car speech recognition model.
Training speech recognition models for in-car environments come with their own set of challenges, primarily due to the unique conditions and dynamics associated with driving. Here are some key challenges faced during the training of in-car speech recognition models:
Acoustic Variability:
In-car environments are acoustically dynamic, with variations in road noise, engine sounds, and external factors. This variability makes it challenging for the model to accurately recognize and interpret spoken commands.
Background Noise:
Cars can be noisy, and background sounds such as traffic, wind, and other environmental factors can interfere with speech signals. Training models to distinguish between relevant voice commands and ambient noise is a persistent challenge.
Varying Driving Conditions:
Driving conditions change rapidly, from city traffic to highway speeds. Adapting the model to work effectively across a spectrum of driving scenarios is essential for consistent performance.
Occupant Interference:
Multiple occupants in the car may simultaneously speak or make noise, creating challenges in isolating and accurately processing individual voice commands. The model needs to account for these overlapping inputs.
Limited Data for Rare Scenarios:
Anomalies like extreme weather conditions, unusual driving scenarios, or less common accents may be underrepresented in training data. Ensuring the model's robustness in these rare situations is critical.
Real-Time Processing:
In a driving scenario, users expect real-time responses. Training models that can process and respond to voice commands swiftly without compromising accuracy is a balancing act.
Multilingual Support:
Users may speak different languages or switch between languages while interacting with the voice assistant. Training models to seamlessly handle multilingual scenarios add complexity to the training process.
Testing:
Testing an in-car ASR-enabled voice assistant for such diverse scenarios of different speeds, acoustic environments, and background noises can be a time-consuming and expensive task.
Acquiring or Collecting Diverse Dataset:
Collecting a diverse and comprehensive dataset that includes all the scenarios and real-life instances can be a time-consuming and expensive matter.
As we can see, except for technical and safety challenges, the most paramount challenge in training a sophisticated in-car voice assistant is collecting a training dataset for it. Now let’s see what diverse features a speech dataset should consist of for in-car voice assistants.
A speech dataset to train ASR-enabled voice assistants should be diverse and should represent all the scenarios that can occur in real life. Which means it should contain examples of speech for all diverse scenarios. So let’s talk about some of them.
To build the in-car voice assistant speech dataset we need to ensure that it contains the speech data in our target language and it covers all the potential accents of our target users. It ensures that our target audience will never fall short while interacting with our in-car voice assistant.
Along with that, we need to ensure that the speech dataset contains speech data and all potential content that includes
Entertainment Commands
●"Play my favorite driving playlist."
●"Skip to the next track."
●"Find jazz music."
Directional Commands
●"Navigate to the nearest gas station."
●"What's the quickest route home?"
●"Avoid tolls on my route."
Climate Control Commands
●"Set the temperature to 72 degrees."
●"Turn on the air conditioning."
●"Increase fan speed."
In-car System Commands
●"Turn on the headlights."
●"Activate windshield wipers."
●"Adjust seat position."
Communicational Commands
●"Call John."
●"Send a message to Mom."
●"Answer the call."
Emergency and Safety Commands
●"Call emergency services."
●"Report an accident."
●"Activate roadside assistance."
General Conversational Commands
●"Tell me a joke."
●"What's the latest news?"
●"How's the weather today?"
Just like any other speech dataset the in-car voice assistant speech dataset should also contain the speech sample across different user profiles. It includes collecting speech data from males and females across different age groups like kids, teens, adults, and elders. This ensures that our speech recognition model works efficiently with all our potential customers across age groups.
Road and driving conditions affect the acoustic environment of the car. This means when a car runs at 60 kilometers per hour it produces more noise compared to 20 kilometers per hour. Along with that outside conditions affect it in the same way. The acoustic environment in a car when the car is on the highway is different compared to when the car is on a busy street.
So we need to ensure that we capture speech data from different outside environments, and outside background noise across all speeds. It is complex to capture speech data at extreme diversification so it is necessary to choose the most important aspects based on research.
HVAC means heating, ventilation, and air conditioning. As we know at different HVAC conditions the acoustic environment of the car is different. So when AC is running on full throttle there is noise because of that. Such different HVAC conditions can affect the accuracy of our in-car voice assistant immensely.
So we must capture our speech data under different HVAC conditions. This means we must have speech commands recorded without AC and with AC at different fan speeds like low, medium, and high.
Windows and sunroof settings also affect the in-car acoustic environment in the same way. So we need to ensure that we have representation of such different car settings as well. That means our speech dataset should contain a significant number of speech commands with open and closed windows and sunroof to make sure our voice assistant doesn’t fall short in such conditions as well.
Having a diverse speech dataset that contains the speech samples recorded in the morning, afternoon, and evening and training our in-car speech recognition model on such a diverse dataset ensure more robustness.
Car environment and noise also affect the speech model accuracy. We can collect speech datasets in the different in-car environments like with music on, with co-passengers talking, etc. Such diversity can make our model ready for all edge scenarios.
From our discussion till now we can understand that collecting and preparing a diverse speech dataset for in-car voice assistants can be complex as it can be time-consuming, costly, and have some safety challenges as well. The key here is to prioritize the things based on the research and focus on that.
We at FutureBeeAI can assist you with collecting the most diverse speech dataset with our global crowd network. With our years of experience and state-of-the-art speech dataset collection and processing tools, we can help you collect the most accurate and high-quality speech dataset with required technical features like sample rate, bit depth, and audio format with required transcription.
Feel free to reach out with all your in-car speech recognition speech dataset requirements and let us help you make it a reality.