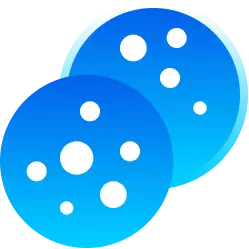
We Use Cookies!!!
We use cookies to ensure that we give you the best experience on our website. Read cookies policies.
A multinational technology company sought to build a robust natural language processing (NLP) model capable of extracting named entities from unstructured text data across multiple languages. Their raw dataset, however, was inconsistent and lacked the structure required for effective model training. The challenge was to preprocess the unstructured data, improve its quality, and perform Named Entity Recognition (NER) annotation with 20 labels, including person names, organization names, locations, product names, and others.
FutureBeeAI provided an end-to-end solution, beginning with a comprehensive quality assessment of the raw data. Our team performed rigorous preprocessing to enhance data consistency, dividing the text into smaller, meaningful sentences for more accurate annotation. Using our global community of linguists and annotators, we annotated 1,500,000 sentences in German, Spanish, French, Arabic, Tamil, English, Hindi, Mandarin, and Tagalog.
Get It Now