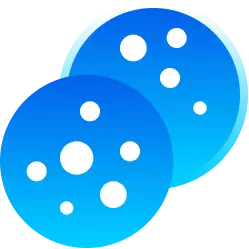
We Use Cookies!!!
We use cookies to ensure that we give you the best experience on our website. Read cookies policies.
Conversational artificial intelligence has established its recognition over the last six to seven years, and it seems like there's an application in all industries. Conversational artificial intelligence (AI) is used to help imitate human interactions by recognizing speech and text inputs, as well as translating their meanings across various languages. This technology is used by chatbots or virtual agents, and it relies on large volumes of data, machine learning, and natural language processing.
So what are conversational AI applications, and what makes them a must-have in our daily lives? In this blog post, we'll cover all that and more about it. So let's just dive into it!
Data Collection is the first and foremost step of any data science project. Machine Learning professionals spend ~30-40% of their time in data collection in any data science project. And to be honest, that is the most daunting but most important task of the whole data science life cycle.
Any machine learning or deep learning model you develop depends on the data you feed it because a model identifies the patterns from your data
Let's take an example to understand this. Suppose your team is working on an object detection model. Your data engineering team has prepared the dataset that includes different classes like person, cat, and dog. Now comes your model development team. No matter how well they develop the model, it won't be able to detect traffic lights because you did not feed data of traffic lights.
So, now that you have understood the importance of conversational ai and data collection. Let's discuss How you can efficiently collect data for your conversational ai project.
Collecting and annotating data for conversational ai products are different than the other ai products. Your conversational ai system should be universally operable. To accomplish this, a process called NLU (Natural Language Understanding) is implemented. The following three are the main concepts used to process these diverse inputs.
The intent is all about knowing How users express their goals and needs. Are they looking for information? How are they commanding it? Whether they are asking questions or making a request? Whether they ask follow-up questions or not? All these aspects help a machine in classifying intents accordingly and thus give a better response.
Anything the user says is an Utterance. For example, if a user says "Show me a Cricket News." The entire sentence is the utterance. The utterance is used to identify the intent of the user. In this example, the user intends to watch cricket news.
The mapping of different utterances and specific goals is known as Utterance Collection. Technically, data annotators work on this.
An entity is a word or phrase which modifies users' intents. In our previous example, "Show me a Cricket News." Cricket is an entity. This denotes that the user wants to see Cricket news and not any other news.
Entity extraction is an information extraction technique to classify the key elements from text into predefined categories.
Though we now know the basics of speech data collection, there are may have some cautions in the machine learning model training operations pipeline.
People from different demographics, ethnicity, and nationalities have different accents, and dictation, and thus training data should consist of people from all possible backgrounds. It's annoying when a chatbot fails to understand our voice.
Thus, your training data should be as diverse as possible to develop a universally operable conversational bot or system. If a model has been trained using data consisting of the American accent only, it would be difficult for a model to understand the Indian accent. It would not just be insulting to your business but also frustrating for your users.
Training data should not consist of developers, executives, and other people close to the project because they introduce a bias in their terminology as they already have an idea about the response to a particular question.
The data collection step is solution compatible. Using the same data for developing products like Alexa, Google Home, etc. (Voice-Based Products) and text-based chatbots is not a good idea. The main reason behind this is that people express themselves differently while speaking compared to texting. We tend to express ourselves shortly and crisply while texting but in a detailed way when we speak.
If you have the ambition to transform your business by developing conversational ai, reach out to us for your data needs. We offer diversified and unbiased speech data, text transcripts, or any other training dataset you may require(🛒Browse our Data Store). Along with that, you may also want to check out our annotation, speech-to-text(Or transcription), and classification solutions.
If you’re someone who wants to earn a couple of extra bucks in your spare time, we have launched our very first android app Yugo, where one can perform simple tasks and get paid for it. Download Now ⚡