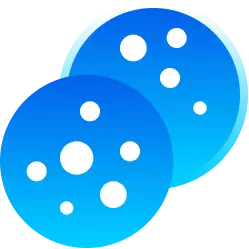
We Use Cookies!!!
We use cookies to ensure that we give you the best experience on our website. Read cookies policies.
If you're a data acquisition manager like me, you know that high-quality data is the key to unlocking the potential of AI/ML and how does AI/ML model learn. It needs data, LOTS of data, powered by human intelligence, and so does the automatic speech recognition model. Without the right data, even the most sophisticated algorithms will struggle to accurately transcribe spoken language. But what makes for quality speech data, and how can you ensure that you're curating the right datasets for your specific use case?
In this blog post, we'll dive deep into the world of custom training datasets for speech recognition, exploring why diverse speech data with different accents, age groups, and speaking styles, along with ground truth transcription, is crucial for developing highly accurate and reliable models. We'll also introduce you to FutureBeeAI, a cutting-edge provider of state-of-the-art platforms for collecting and transcribing high-quality speech data.
With our five-step process, we make it fast and easy to curate custom training datasets that meet your unique needs. We onboard language experts and transcriptionists to our intelligence community, who work with our Yugo platform to streamline the speech data collection process. With our quality control measures and delivery system, you can rest assured that your final dataset will meet your specifications and help you develop the best possible speech recognition models.
So whether you're a speech recognition engineer or a data acquisition manager looking to take your models to the next level, this blog post is for you. By the end, you'll have a firm grasp of the importance of custom training datasets for speech recognition and how FutureBeeAI can help you achieve your goals.
Training data for speech recognition is a dataset used to train machine learning models that can recognize speech and convert it into text.
The audio recordings in the dataset are typically collected from a wide range of sources and are designed to represent the full range of speech patterns and accents that the speech recognition system is intended to recognize. These audio recordings may include speeches, conversations, and another spoken language, and they may be collected from diverse populations with different accents, dialects, and speaking styles.
The transcriptions for each audio recording are usually created manually by humans or using automatic speech recognition tools, and they are designed to accurately represent the spoken language in the audio recording. These ground truths are used to train the speech recognition models to recognize patterns in the audio signals and convert them into text.
In addition to the audio recordings and transcriptions, speech recognition training data may also include additional metadata, such as speaker information, recording quality information, and information about the recording environment. This metadata can help to improve the accuracy and effectiveness of the speech recognition system.
One can find a lot of speech data available as open source to develop a speech recognition model but when it comes to developing a high-quality and use case-specific model, needs custom training data as per the requirements of the given use case.
So, let’s understand the custom training dataset.
A custom training dataset for speech recognition is a dataset that is tailored to a specific application or use case.
Let’s understand this with an example, A bank wants to use speech recognition technology to automatically transcribe calls to their customer support center. This could help identify common issues and concerns among customers, allowing the bank to proactively address these issues and improve customer satisfaction.
For this use case, we must have to collect recordings of customer interactions that represent the range of speech patterns and accents that the speech recognition system is likely to encounter. This may involve recording calls from a variety of different regions or countries and speakers with varying accents.
In this call-center data, we will find that this is a formal conversation between customer and agent that may have different outcomes positive, negative, and neutral. These patterns are very important for an ASR model that is built for a banking use case and you may not find this type of data in open source or open source data may lack the vocabulary used in the banking sector.
After collecting a custom dataset we have to do a transcription of these audios to make it usable for an ASR model.
Transcription is the process of converting spoken words or audio recordings into written text. This can be done manually by a skilled human transcriber or through automated transcription software.
To increase the accuracy of speech models we also include speaker tags and background environment labels within the transcription and that is why we also called this audio annotation.
Transcription = Ground Truth of Audio
Both Speech data and Transcription are very important for an ASR model to get trained and become a highly accurate AI model. Speech data collection and manual transcription take time and expertise and you should rely on a trustable partner for these datasets.
We at FutureBeeAI understand that building ASR models is an eventful process and needs accurate datasets. We have made the training data collection process fast-paced with the help of our state-of-the-art platforms including the speech data collection platform and manual transcription platform Yugo.
For us, it is a 5 step process to collect high-quality custom training for your ASR needs but it is only 1 step for you. Your only one step means you just need to connect with FutureBeeAI to tackle your data needs.
FutureBeeAI’s 5 Steps = Ready to Deploy Custom Training Datasets = Your 1 Step
Our first step in any custom training data collection is to understand the use case and decide on the target language, accent, community, age group, format, quality, quantity, etc.
In this step we thoroughly discuss the use case, check all the different scenarios, the purpose of the model, the scope, the diversity of data, and ethical considerations.
All these things are very important to build a world-class accurate ASR model. By discussing all these points we the idea about the actual needs and it helps us to serve better.
This is our 2nd and most crucial step to start any training data collection. In the 3 years, we put some good efforts into building a community that is intelligent and understand the importance of accuracy when it comes to developing AI models. Similar to the AI model, continuous training is the key for our community to become intelligent.
According to the given use case we onboard language experts, domain experts, and skilled transcribers. Before they start the actual work, we make them understand the use case, and provide training for specific requirements. The good thing here is we have our Speech collection and transcription platform that can be used in all use cases and is very easy to use having our platform save us a lot of time as our community does not need to work on different platforms every time.
So, onboarding done, let’s start flying high.
This is the soul and heart step of our data collection process. This is where the preparation of the dataset starts. Depending on the requirement we launch projects through our Speech data collection platform Yugo.
Yugo speech is capable of collecting prompts, wake words, and conversation recordings between two people. For prompts and wake words we upload scripts to be recorded and for recording free-flow conversations we launch separate projects where two people can join from different places and talk.
In the output, we will get speech data with proper format and metadata. Once the data is recorded and get reviewed by our quality team goes to the Yugo Transcription platform.
Once we get the high-quality speech data, parallelly we can start the transcription. Here we can assign different audios to expert transcribers from our intelligence community. The final output will be in JSON format.
The pack is ready, Speech+Ground Truth. Let’s check the quality.
As we have successfully collected the speech data and done with transcription, we must have to add a quality check layer to avoid quality issues that can deteriorate the speech model’s accuracy.
In this step we involve our language-specific dedicated team to check the output thoroughly and make sure that everything is as per the requirement and ground truth.
Oh wow, the data is good and meets the client’s requirements let’s deliver this.
We are on time, yes we deliver all the requirements to our clients on time. This is a fearless step for us as we have gone through four very important steps. Here we will give the final touch by again checking format, volume, and data distribution.
In this step, we deliver the output in batches and through the cloud. We collect and deliver all data through high-secure cloud storage platforms like AWS and Microsoft SharePoint.
Data is ready, I mean ready to deploy.
Days are near when we talk to fluent machines, and for this milestone, we should thank human intelligence because machines can not learn without high-quality training data. AI models need custom training data for different use cases and key partners like FutureBeeAI having intelligent community and state-of-the-art platforms that support data collection are very important to make machines more intelligent!